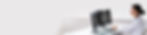
Detection of suspected cerebral aneurysm sites from brain MRA images
Using artificial intelligence (AI) technology, saccular unruptured cerebral aneurysms and multiple cerebral aneurysms are detected and displayed as bounding boxes.
In addition, non-benefit claims are possible with innovative medical technologies that have proven safety and effectiveness that can be used in medical settings.

Eligible for non-covered service
Designated as an innovative
medical technology
Ver.2.0.0
DN-CA-01
Approval from the Ministry of Food and Drug Safety (No. 20-467)
Indicate the location of the suspected cerebral aneurysm
Sensitivity
87% More than
Specificity
92% More than
91.11%
93.91%
Ministry of Food and Drug Safety Clinical Trial Results
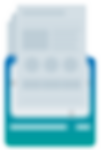
1. AI analyzes medical image data related to each disease and assists medical institutions and doctors in reading.
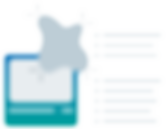
2. Quickly locate the disease
Detects and alerts you to anything suspicious.
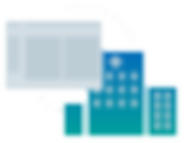
3. Easy PACS interworking / It is interlocked with the PACS in the hospital through simple DICOM communication.
AI-informed Treatment Decisions in Minutes
Changes that DEEP:NEURO will bring

Accuracy
Increase diagnostic accuracy by marking the suspected cerebral aneurysm area with a bounding box on brain MRA images.

Efficiency
Reduces the burden on medical staff's interpretation work due to increased examination volume and improves diagnostic efficiency.

Point of Care
It prevents lesion omission and contributes to securing the golden time for patient treatment.
Publications
-
So Yeon Won, et al., “Reproducibility for carotid wall segmentation using T1-weighted DANTE-SPACE sequence on high-resolution 3-T carotid MRI”, Acta Radiologica, 2024.
-
Min-Seok Kim, et al., “Deep-Learning-Based Cerebral Artery Semantic Segmentation in Neurosurgical Operating Microscope Vision Using Indocyanine Green Fluorescence Videoangiography”, Frontiers in Neurorobotics, 2022.
-
Bio Joo, et al., “A Deep Learning Model with High Standalone Performance for Diagnosis of Unruptured Intracranial Aneurysm”, Yonsei Medical Journal, 2021.
-
B. Sohn, et al., “Deep Learning–Based Software Improves Clinicians’ Detection Sensitivity of Aneurysms on Brain TOF-MRA”, American Journal of Neuroradiology, 2021.
-
Seung-Yeon Lee, et al., “Performance evaluation in [18F]Florbetaben brain PET images classification using 3D Convolutional Neural Network”, PLOS One, 2021.
-
Bio Joo, et al., “A Deep Learning Algorithm May Automate Intracranial Aneurysm Detection on MR Angiography with High Diagnostic Performance”, European Radiology, 2020.
-
Younghee Yim, et al., “Pretreatment brain volumes can affect the effectiveness of deep brain stimulation in Parkinson's disease patients”, Scientific reports, 2020.
Conference Abstracts
-
Kwanseok Oh, et al., “Quantitative Evaluation of the Deep Learning-Based Aneurysm Localization and Its Size Measurement Solution on Brain Magnetic Resonance Angiography”, KCR, 2024.
-
Kwanseok Oh, et al., “Context-aware Semantic Augmentation for Semantic Segmentation-based Intracranial Aneurysms Detection for Various Sizes”, KCR, 2024.
-
Bio Joo, et al., “Deep learning-based automated detection and localization of cerebral aneurysms on MR angiography”, RSNA, 2019.
-
Bio Joo, et al., “Deep learning-based automated detection and localization of cerebral aneurysms on MR angiography”, KCR, 2019.
Research Activities
-
Hyung Eun Shin, et al., “Brain Tumor Segmentation using 2D U-net”, BraTS, 2018.
-
Hyung Eun Shin, et al., “Identifying the Best Machine Learning Algorithms for Brain Tumor Segmentation, Progression Assessment, and Overall Survival Prediction in the BRATS Challenge”, BraTS, 2018.